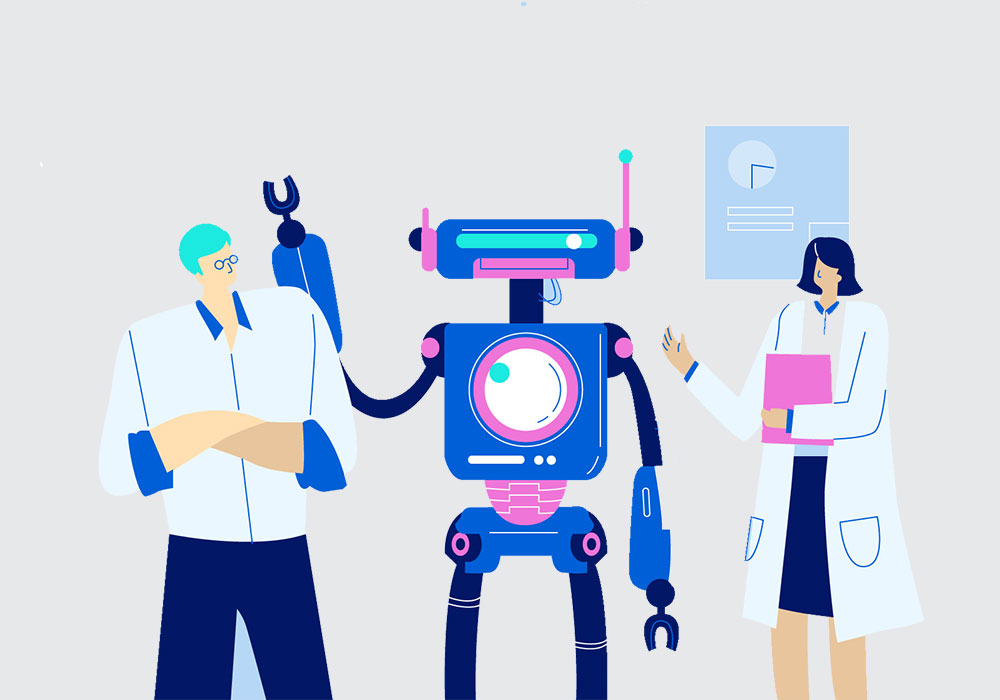
Machine Learning in Supply Chain and Logistics
Machine Learning is transforming supply chain management by optimizing demand forecasting, route planning, inventory management, and predictive maintenance. Time-series forecasting models such as ARIMA, Prophet, and LSTM networks analyze historical sales data to predict future demand, enabling businesses to manage inventory efficiently and reduce stockouts.
In logistics, route optimization is achieved through algorithms that analyze real-time traffic data, weather conditions, and delivery schedules. Reinforcement Learning is particularly effective in dynamic route planning, continuously learning from new data to improve delivery times and reduce fuel consumption. Companies like UPS and FedEx use ML-driven logistics systems to optimize their global operations.
Inventory management benefits from predictive models that forecast demand fluctuations, optimize stock levels, and automate replenishment processes. ML algorithms identify patterns in sales data, supplier performance, and seasonal trends to ensure the right products are available at the right time.
Predictive maintenance is another critical application, where ML models analyze data from IoT sensors embedded in machinery and vehicles. By identifying early signs of equipment failure, businesses can perform maintenance proactively, reducing downtime and maintenance costs. Algorithms like Random Forests, Support Vector Machines, and Neural Networks are commonly used in predictive maintenance systems.