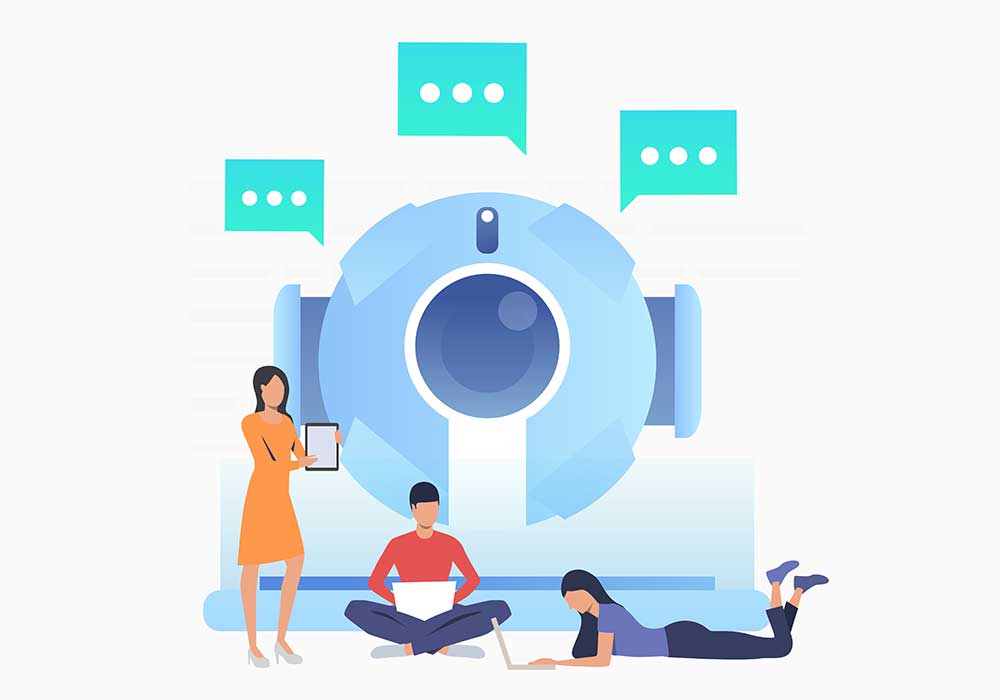
Machine Learning in Healthcare
Machine Learning (ML) has significantly transformed the healthcare industry by enabling faster, more accurate diagnoses, optimizing treatment plans, and predicting patient outcomes with high precision. One of the most impactful applications is in medical imaging, where algorithms like Convolutional Neural Networks (CNNs) analyze X-rays, MRIs, and CT scans to detect anomalies such as tumors, fractures, and internal bleeding. These models can identify patterns in medical images that may be too subtle for the human eye, improving early detection rates for diseases like cancer.
Moreover, predictive analytics in healthcare leverages historical patient data to forecast potential health risks. For example, models based on logistic regression or gradient boosting predict the likelihood of heart disease, diabetes, or stroke by analyzing patient history, genetic data, and lifestyle factors. Natural Language Processing (NLP) is another critical ML application, used to process and extract meaningful insights from unstructured data in Electronic Health Records (EHRs). NLP helps identify key medical conditions, recommended treatments, and patient trends, supporting clinical decision-making.
Furthermore, personalized medicine is becoming more feasible through ML. By analyzing genetic data and patient-specific factors, ML models recommend tailored treatment plans that optimize drug efficacy and minimize side effects. In drug discovery, reinforcement learning and deep learning algorithms accelerate the identification of potential drug candidates, significantly reducing the time and cost involved in bringing new drugs to market.